주제를 살펴보자 “3d vision – Lecture 17: 3D Vision” 카테고리에서: Blog MMO 에 의해 컴파일 Ko.nataviguides.com 다양한 소스에서. 저자별 기사 Michigan Online 가지고 조회수 13,346회 그리고 의지 좋아요 230개 높은 평가.
이에 대한 추가 정보 3d vision 주제에 대해서는 다음 문서를 참조하십시오. 아이디어가 있으면 기사 아래에 댓글을 달거나 주제에 대한 다른 관련 기사를 참조하십시오.3d vision 관련 기사 섹션에서.
Table of Contents
주제에 대한 비디오 보기 3d vision
아래는 주제에 대한 자세한 비디오입니다 3d vision – Lecture 17: 3D Vision. 계속 주시하고 읽고 있는 내용에 대한 피드백을 보내주세요!
Lecture 17: 3D Vision – 3d vision 및 이 주제에 대한 세부정보
주제에 대한 설명 3d vision:
Lecture 17 discusses ways for incorporating 3D structure into neural networks. We talk about different representations for 3D data including depth maps, voxel grids, implicit functions, point clouds, and triangle meshes. We show how graph convolution is a neural network primitive that respects the structure of triangle meshes. We discuss metrics for comparing 3D shapes including intersection over union (IoU), Chamfer distance, and F1 scores. We briefly talk about camera systems, and specifically the difference between canonical and view-centric coordinate systems. We discuss datasets for 3D shape prediction including ShapeNet and Pix3D, and discuss the Mesh R-CNN architecture for performing joint object detection and 3D shape prediction.
Slides: http://myumi.ch/jxDrl
_________________________________________________________________________________________________
Computer Vision has become ubiquitous in our society, with applications in search, image understanding, apps, mapping, medicine, drones, and self-driving cars. Core to many of these applications are visual recognition tasks such as image classification and object detection. Recent developments in neural network approaches have greatly advanced the performance of these state-of-the-art visual recognition systems. This course is a deep dive into details of neural-network based deep learning methods for computer vision. During this course, students will learn to implement, train and debug their own neural networks and gain a detailed understanding of cutting-edge research in computer vision. We will cover learning algorithms, neural network architectures, and practical engineering tricks for training and fine-tuning networks for visual recognition tasks.
Course Website: http://myumi.ch/Bo9Ng
Instructor: Justin Johnson http://myumi.ch/QA8Pg
자세한 내용은 Lecture 17: 3D Vision 의견 섹션에서 또는 주제에 대한 다른 기사를 확인하십시오 3d vision.
키워드 정보 3d vision
아래는 에서 검색한 결과입니다 3d vision 빙 사이트에서. 원한다면 더 읽을 수 있습니다.
기사 3d vision – Lecture 17: 3D Vision 우리는 인터넷의 다양한 소스에서 편집합니다. 이 기사가 유용하다고 생각했다면. 더 많은 사람들이 볼 수 있도록 공유로 응원해주세요! 감사해요!
콘텐츠에 대한 이미지 3d vision
주제에 대한 사진 Lecture 17: 3D Vision 항목의 내용을 더 잘 이해할 수 있도록 항목을 설명하는 데 사용됩니다. 필요한 경우 댓글 섹션이나 관련 기사에서 더 많은 관련 사진을 찾을 수 있습니다.
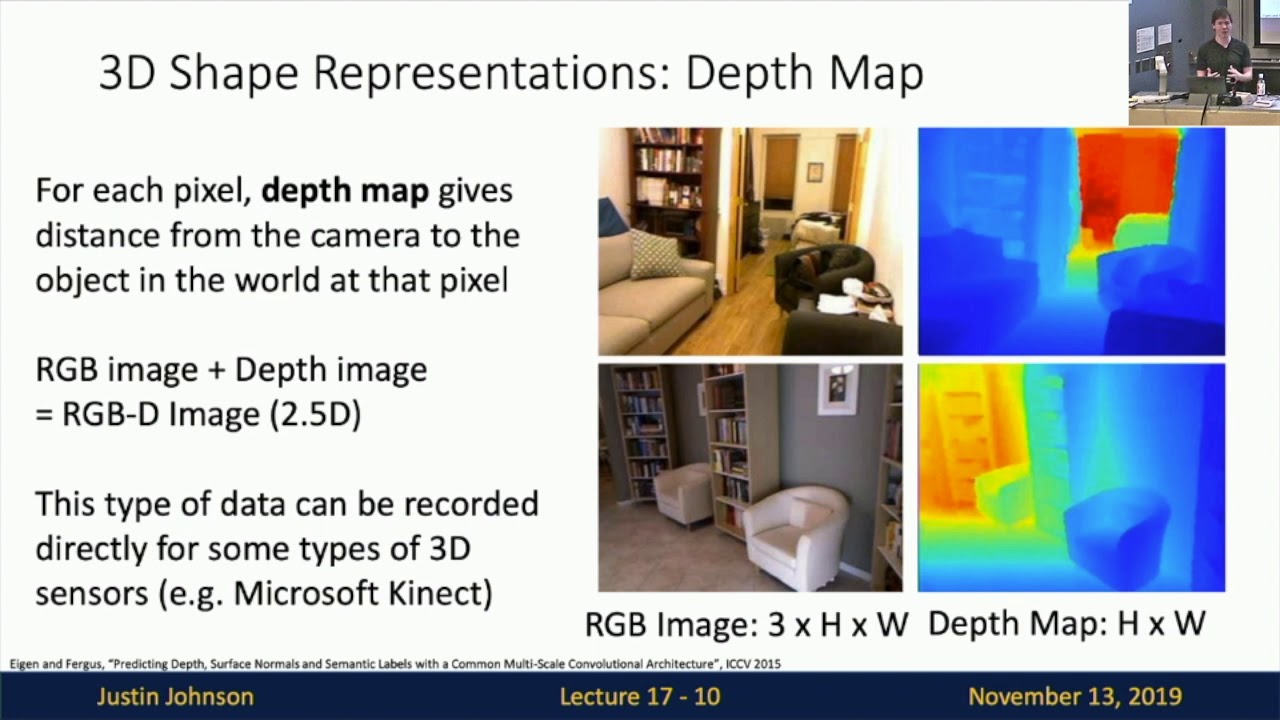
주제에 대한 기사 평가 3d vision
- 작가: Michigan Online
- 보다: 조회수 13,346회
- 좋아요 수: 좋아요 230개
- 동영상 업로드 날짜: 2020. 8. 10.
- URL 비디오: https://www.youtube.com/watch?v=S1_nCdLUQQ8
기사의 키워드 Lecture 17: 3D Vision
- Michigan Online
- Online Learning
- Education
- 3D vision
- shape prediction
- depth maps
- RGB-D
- voxel grids
- implicit functions
- OccNet
- point clouds
- PointNet
- Chamfer distance
- triangle meshes
- Pixel2Mesh
- graph convolution
- intersection over union
- IoU
- F1 score
- ShapeNet
- Pix3D
- Mesh R-CNN
Lecture #17: #3D #Vision
주제에 대한 더 많은 비디오 보기 3d vision 유튜브에
또한 더 많은 키워드 뉴스를 보려면 최신 뉴스레터를 확인하십시오 Lecture 17: 3D Vision 보다.
방금 주제에 대한 기사를 읽었습니다 3d vision – Lecture 17: 3D Vision. 이 기사의 정보가 유용했다면 공유해 주세요. 매우 감사합니다.